In 2016 a company calculated that the average number of followers in Twitter was about 707. Although this might already sound like a lot, it pales in comparison with the top Twitter users. There are currently more than 100 users that have more than ten million followers. Some, like Barack Obama or Katy Perry, even have more than one hundred million followers, which is roughly 140.000 times larger than the average.
When the average number of connections in a network is not representative for the many large values we observe, network scientists call the network scale-free. This term reflects the fact that there is no typical scale. Many nodes have far more connections than the average suggests. The vast research on scale-free networks and their properties has helped make network science become the prominent research field it is today.
However, a cumulating lack of understanding and misconception among scientists regarding scale-free networks has led to confusion what scale-free networks are and if they are as omnipresent as earlier research suggested.

Visualisation of a scale free network
This problem is currently shaking the foundation of many branches of network science. Much research on networks has been based on the scale-free property. If most networks would turn out not to be scale-free then these results would end up in the garbage, setting network science back decades.
So what are scale-free networks exactly, what is the problem with them and most importantly, how can mathematics help resolve this?
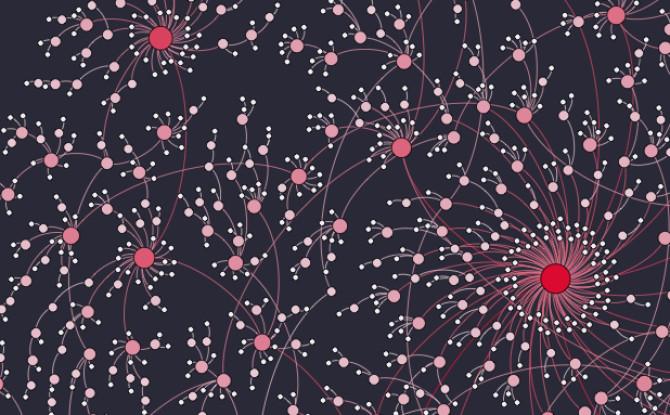
Artistic visualization of a scale-free network.
No typical users in Twitter
Let us go back to the example of Twitter. This is a social network, a place where people interact and share information and opinions. When network scientists look at Twitter they also see a network, but in a more scientific way. For them a network is an abstract object made up of nodes and connections between them. In some cases these connections can be symmetric, like friendship relations. Other times they can have a direction. In the Twitter network, nodes are users and there is a directed link pointing from one user to another if the first user follows the second one. If you follow Barack Obama, then there is an link pointing from you to him. The number of followers a user has is called its degree. Users with many followers, such as Barack Obama and Katie Perry, have very large degrees and are called hubs.
The world around us is littered with many different types of networks. There are other social networks such as Facebook or Snapchat. Technological networks like the World Wide Web, consisting of webpages and hyperlinks. You probably used this network to find this article. Even the collection of intricately connected neurons that make up our brain form a network. Although these networks describe different systems, researchers have found that many of them contain hubs whose degree is several orders of magnitude larger than the average degree in the network. Think about how many friends/fans Katie Perry must have on Facebook or how many webpages have a link to the Wikipedia page of Stephen Hawking.
Why there are no real giants on earth
This extreme variety in the degrees of nodes is quite different from many other quantities we encounter in our everyday lives. For example, the tallest person in the world, Sultan Kosen, is 251cm. Although he might seem like a giant compared to the average Dutch male, whose height is 186cm, he is not even twice as tall. Imagine the heights of people would be like the number of followers in Twitter. Then the tallest person in the world would be approximately 260km!
The absence of extremely large people can be observed mathematically, by looking at how the heights of people are distributed. Like many other every-day quantities, this distribution closely resembles what we call a normal distribution. This distribution is characterized by a symmetric curve, whose peak is at the average value and which quickly decreases when we move away from this value (see top picture on the left). The first part means that the height of most people will be very close to the average. The second part tells us that, although there are people that are reasonably smaller or larger than average, it is very unlikely to see values that are really far from the average. There are no giants among us.
Why there are giants in Twitter
For the number of followers in Twitter the story is different. Researchers found that this distribution is not a normal distribution but exhibits a so-called power-law behavior. In fact, many other networks were found to have a degree distribution which resembles a power-law. Unlike the normal distribution, power-law type distributions are not centered around their mean and decrease much more slowly (see the bottom plot in the figure above). This increases significantly the probability to see, for example, users with a large number of followers or webpages with many links.
The number of followers in Twitter has a power-law behavior
How fast power-law distributions decrease can be characterized by a number, called the power-law exponent. This exponent is always greater than 2, and the larger it is, the quicker the distribution decreases. In other words, the larger the exponent, the smaller the variation in the degrees. An important situation occurs if the exponent is between 2 and 3. In this case the mean of the distribution is finite, but the variance is infinite. This means that although the average degree is some finite number, we are expected to see extremely large deviations from this value.
For Twitter the exponent is estimated to be around 2. This agrees with the existence of giants, like Barack Obama and Katie Parry, with more than one hundred million followers while the average number of followers is only 707. We must conclude that the average number of followers in Twitter is by no means a good indication for the number of followers we can expect to see. The existence of such extremely large deviation from the average degree implies that there is no typical scale for the degrees. That is why network scientist referred to such networks as scale-free networks.
Choosing between a rock and a hard place
The real problem is that this disagreement about what scale-free networks are and how to test if a network is scale-free can have far reaching consequences. Suppose, for example, that we want to use network analysis for designing effective strategies to combat the spreading of misinformation. Since the way information spreads in networks depends on the existence of large hubs, so does the choice of the right strategy. Thus it really matters if the network on which the misinformation spreads behaves like a scale-free network or not. If one group of researchers claims this is not the case, using this new restrictive definition, while another claims it is, based on a non-rigorous definition, then what should we do?
There are two obvious choices. However, each of them has the potential to undo years of research on networks. If we choose to stick with the restrictive definition we will likely conclude that scale-free networks are rare and as a consequence lose many results regarding the behavior of networks. This actually does not have to be true, since the behavior observed for scale-free networks could remain valid if we consider other, less restrictive, definitions. On the other hand, if we decide to continue with classifying scale-free networks using intuitions that lack a rigorous foundation we can end up making claims about the behavior of networks that are wrong.
To really resolve this problem, we need a proper definition of power-law degrees and scale-free networks. But how should we define these notions in such a way that it is maximally inclusive in capturing the associated intuitions and still allows us to estimate their presence among networks in the real world?
Extreme event mathematics
This is where mathematics enters the stage. More precisely the field of mathematics that studies extreme events. These are events that do not occur often but can have a large impact, such as earthquakes and tsunamis, or the resulting very large claim amounts with insurance companies.
We can think of seeing nodes with large degrees as such an extreme event; there are not that many of them but they can have a significant impact on the network.
A key tool in this field of extreme-value theory is the so-called Extreme Value Index (EVI) of a distribution. This index can be estimated from the data and tells you how large the extreme values can actually be. For example, the maximal strength of an earthquake or the largest claims an insurance company is expected to receive. In the context of Twitter, extreme events can correspond to observing a user with a very large number of followers.
Using extreme value theory we can properly define scalle-free networks
From the depths comes a solution
The connection between the Extreme Value Index and power-laws comes from a deep result in extreme-value theory. This result tells us that the distributions with positive index behave like power-laws, in a way that corresponds with the long standing intuition of network scientists. More precisely, distributions with positive index belong to the class of so-called regularly varying functions. This class includes the restrictive definition used to conclude that scale-free networks are rare, but contains many other functions which have similar mathematical properties.
Distributions with positive EVI are thus the best definition for capturing the intuition network scientists have concerning power-law degrees. In addition, the EVI is directly related to the power-law exponent, which was so crucial for the behavior of networks. Therefore, by estimating the EVI of degree distributions in networks we can solve both problems. We say the degrees behave like a power-law if the EVI is positive and call the network scale-free precisely when the corresponding estimated exponent is between 2 and 3.
Finally, since estimation techniques for the EVI have been in development since the 1950’s, a variety of powerful and precise estimation tools are readily available to use. This way, mathematics gives us a powerful notion of a scale-free network, using the right combination of a broad but precise definition, while at the same time providing researchers with tools to test this on real-world networks.
It is as if we can give those two carpenters a mathematical definition of a chair that captures both their ideas, as well as a machine that can tell them how good the chair in front of them is. The Extreme-Value approach to scale-free networks will aid networks scientist in engaging in productive scientific discussions on the topic. This will create a better understanding of scale-free networks and their behavior, while still being able to rely on known results. Thus saving decades of network research from the trash bin.
The visualizations of the scale-free networks were made by Yuntao Jia, Jared Hoberock, Michael Garlang and John Hart and published in "On the Visualization of Social and other Scale-Free Networks". The featured image was made by P.J. Lamberson and can be found here.[