What does a working week, vacation, board games, football and faulty machines all have in common? In the not so distant future…
After a busy week at work, Reinforcement Learning slowly sinks into his leather armchair. A smoky aroma fills the room to the tune of a crackling fire. With a deep gaze fixed on the flames dancing in the fireplace, he leisurely takes a sip of red wine. He cherishes these moments where he can simply relax, take a pause and feel the heat of the flames on his sun kissed cheeks. However, this moment is short lived as his attention is drawn to his favourite evening activity. His gaze slowly moves towards a large ledger lying on a table next to his armchair. This ledger contains detailed accounts of instances where he helped solve a variety of real world problems. He lazily picks up the heavy journal, places it on his lap and starts to trace over the corners of the pages with his right thumb. As his thumb grips each corner of a page a familiar sound, similar to that of folding two decks of playing cards, rifles through the air. Before reaching the last page, he comes to an abrupt stop. With his thumb he divides the journal at the arbitrarily chosen pages. The journal falls open on his lap and he starts to read out loud…
We are all living in the age of information, where words like internet of things, big data and artificial intelligence has gained a lot of traction. One of the leaders standing at the forefront of innovation is Reinforcement Learning. The foundational principal of Reinforcement Learning comes quite natural to us humans. It is based on the idea of learning from the environment by means of interaction, just like we predominantly did when we were toddlers. However, instead of pulling on table cloths to see what happens, Reinforcement Learning learns from a mathematical or data driven environment. Better yet, he learns without any parental supervision!
A broad smile spreads across Reinforcement Learning’s face, as these words took him back to his beginning. He was not conceived in the traditional sense, but rather born from an idea in the late 1950s. Richard Bellman derived a set of equations, while working on control theory, and unknowingly set into motion a chain of events leading to Reinforcement Learning’s emergence. Looking back, it is easy to connect the independent dots which made Reinforcement Learning the man he is today.
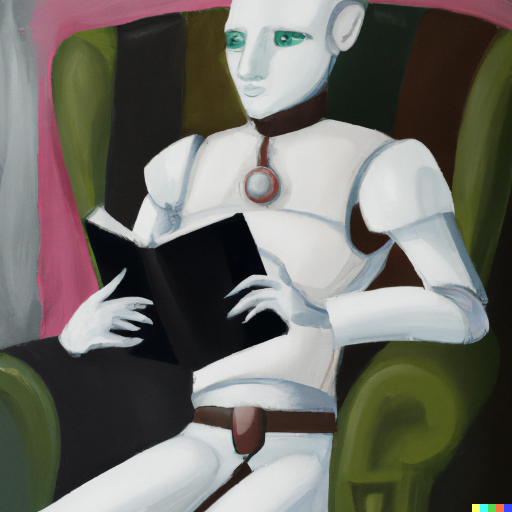
As Reinforcement Learning admired the ruby tint of the playful flames through the wine glass, he recalled his fondness for board games. He paged through his journal, past AlphaGo, AlphaGo Zero and Atari, where he defeated professional human players at each game respectively. He stopped paging as soon as he reached his favourite game… planning a vacation. At the end of the day, most things in life can be seen as a game and it is all about how you play it. Reinforcement Learning enjoys his travels in a similar fashion than he conducts his daily work, i.e. making decisions under uncertainty.
Like any normal person, his vacation would always start at home. Unlike most people, his next destination was decided by chance. He would throw a special dice to determine where to go. After arriving at a holiday destination, he could decide to either go home or throw the dice again. Holiday destinations included Egypt, the Amazon rain forest, Disney World, a swamp, the sewer, etc. (some more glamorous than others). The aim of his vacation game was simple: Organize the best possible holiday! After going on multiple vacations, Reinforcement Learning gained insight on whether or not to go home after visiting specific holiday destinations. He quickly realized that occasionally the road to Rome leads through a swamp. However, often the happiness gained from going home outweighed the misery of ending up in the sewer. By taking into account the experience he gathered from previous vacations, he could use mathematical techniques to learn when to go home and when to throw the dice and go to the next holiday destination.
This train of thought brings Reinforcement Learning to one of the earliest forms of games, namely sport. In the past, playing physical sports has never been an area Reinforcement Learning excelled at. Even though he is an able-bodied man, he usually stumbled onto the field with two left feet. Therefore, Reinforcement Learning usually stayed on the sidelines and mainly focused on providing tactical insights, for both players and coaches.
A large part of the journal’s sport section is dedicated to football implementations. The mechanics of a football game can simply be described as a team of players following strategies in order to score more goals than their opposing team. When a player has the ball at their feet, they need make a decision. These decisions include passing, dribbling or shooting and each decision has a success rate, which depends on the skill level of the player and their opponent. The coach is responsible for finding a combination of suggested decisions for all the players. This combination is called a tactic and should ideally lead to the team winning the match. Therefore, both the coach and the player needs to make decisions under uncertainty during the game. In the past, Reinforcement Learning has helped with this decision making by considering both mathematical football models as well as player statistics. He learned by observing each player’s ability and how each decision influenced the outcome of the game. This not only helped Reinforcement Learning formulate a winning tactic, but also gave him insight on where to strengthen the team.
Reinforcement Learning was mainly restricted to a football field of zeros and ones. Fortunately, with the help of enthusiastic and supportive humans, he was able to make a lot of progress in the virtual world. These advances includes running, boxing and even driving and parking cars in simulated environments. He continued making progress up until the day he was able to break free from the virtual world. Reinforcement Learning fondly remembers taking his first steps outside of the computer. His unsure movements mimicked that of a newly born giraffe. Luckily, his human friends were always there to lend a stable hand.
As the night grew older, Reinforcement Learning flipped tiredly through the pages. In these last few journal entries, he played an important role in maintaining infrastructures and means of transportation. Often in maintenance practice, seemingly simple decisions need to be made to improve the efficiency of complex systems. For Reinforcement learning, a powerful example that springs to mind is aircraft maintenance. Here, maintenance policies usually boil down to the question: should there be maintenance done at a given time? The format of the answer is simple, yes or no, but the outcome of the answer is crucial as it needs to ensure that the aircraft stays in the sky. A wrong answer could have catastrophic consequences. Systems, such as aircraft, consist of multiple underlying components, and mathematically modeling such systems is immensely difficult due to the correlation and dependencies between
components.
For instance, aircrafts are comprised of multiple connecting parts all working together in mechanical harmony to ensure flight. These parts require attentive maintenance policies; however, due to the dependencies between parts, a vibration in one part could lead to quicker deterioration of a crucial component and the cumulative results could be costly or even catastrophic. Additionally, the exact lifespan of a component is uncertain, which further increases the difficulty of modeling these systems. In such a case it is particularly challenging to determine maintenance policies due to uncertainty and intricate mathematical modeling requirements between parts. Reinforcement Learning assisted with the development of maintenance policies which incorporated the condition of the aircraft as a whole. After observing a few flights and maintenance schedules, Reinforcement Learning gained a deeper understanding of the problem. He had insight into which replaced parts signaled problems elsewhere in the aircraft, which he used to determine new maintenance policies.
As the flames start to tire and the red wine starts to stain, Reinforcement Learning drifts off to sleep again. Whom will call upon him for help next? Will he be used in finance, health care, robotics, self-driving cars, etc. The main limitation to his applicability is the creativity of his users.
The image in this article was generated using DALL-E 2.